Today, data has become a source of real vitality for businesses due to the constant advancement of technology. Why is data management important to an organization? Data management plays a crucial role in organizations for several reasons. Data provides valuable insights and expands growth opportunities. Managing data effectively ensures its accessibility and security, with proper data enabling organizations to fully utilize their resources. In sectors such as manufacturing, industry, logistics, and others, efficient data management brings a range of advantages:
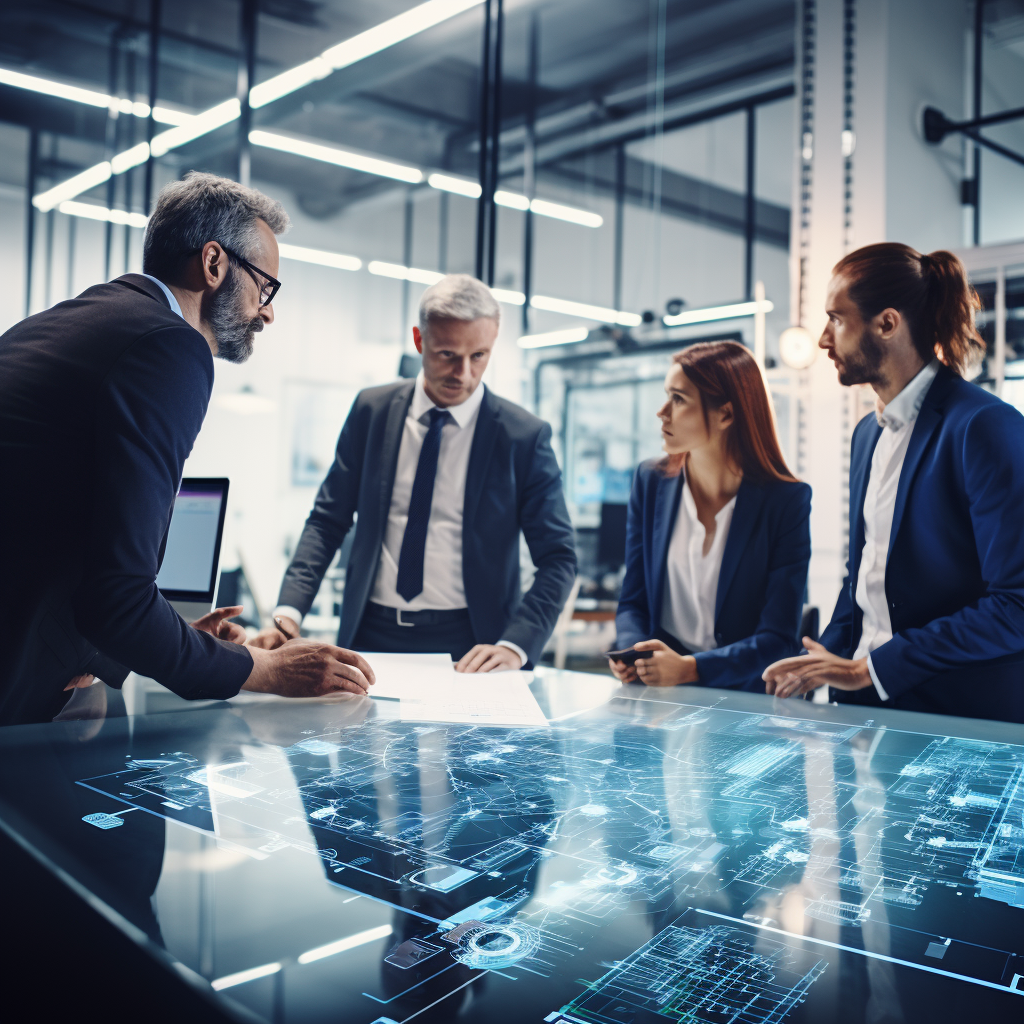
- Data management in manufacturing helps establish strict access controls, encryption mechanisms, and storage policies, thereby safeguarding data from unauthorized access and potential breaches.
- Data management fosters a culture of collaboration by eliminating scattered data repositories, enabling teams within the organization to seamlessly access and exchange necessary information.
- Through data analysis of customer information, companies can personalize services, offer timely updates on supplies, and actively address issues, making it easier to focus on trust and loyalty.
This serves as an excellent solution for optimizing various processes.
Enterprise Data Management Problems and Solutions
We propose to consider the challenges and solutions in data management using financial companies as an example. “Recent studies show that around 62% of financial institutions plan to expand or modify their reference data management strategies within the next two years,” says Mark Brunelli, the chief editor of the news information portal SearchDataManagement.com.
In collaboration with Inside Reference Data Magazine, an IT vendor survey conducted by iGATE Patni revealed that financial organizations are primarily doing this to improve the overall quality of corporate data and reduce the risk of penalties for violating regulatory requirements. So, what are the challenges of data management:
Inefficient Budget Utilization
Many companies spend a significant amount of money on acquiring reference data but allocate insufficient funds to maintain their quality, consistency, and usability. Purchasing the same information for different purposes across various departments is an example of inefficient budget utilization and an ineffective enterprise data management strategy.
Fred Cohen, Vice President and Head of Capital Markets and Investment Banking Practice at iGATE Patni says that some companies spend up to $200 million annually on acquiring reference data, with about $50 million going to waste.
Solution: Implement continuous monitoring of infrastructure. Effective DevOps and DataOps practices help monitor services and resources used for data storage and management. This helps identify cost-saving opportunities, balance scaling expenses, and consider costs early in the data processing pipeline creation. Choose cost-effective tools that align with your budget. Most cloud services are based on pay-as-you-go models, meaning expenses are directly tied to the services and computing resources used.
Unmanageable Volume
Companies deal with massive amounts of data that can easily spiral out of control without proper management. Without the appropriate architecture, computing power, and enterprise infrastructure, they struggle to keep up with data growth and miss out on opportunities to leverage their informational assets.
Solution: Whether choosing cloud, on-premises hosting, or a hybrid approach, ensure that your selection aligns with business goals and organizational needs. For instance, on-premises hosting may not scale instantly and might require physical infrastructure expansion and administrative teams. Still, it’s a suitable choice if you want to maintain complete control over your data. Create scalable architectures and tools that can adapt to the growing data volume without compromising integrity.
Poor Data Quality Leading to Subpar Results
This big data problem costs the U.S. alone over $3 trillion annually. Low data quality leads to errors, reduces efficiency, and results in incorrect conclusions, ultimately transforming into business costs.
Solution: Hire professionals to take care of data in the organization. Define the tools, procedures, and access controls. Set up effective data cleaning, filtering, sorting, enrichment, and other industrial data management processes using modern tools. Understand how to sort and enrich data following specific business objectives. Involving business stakeholders who will use the data might be necessary to determine data quality requirements.
Data Management Vs Business Intelligence Vs Big Data
Business data management involves storing, organizing, and maintaining data in a structured and secure manner to ensure its accuracy, accessibility, and reliability over time. It encompasses data quality management, integration, security, and lifecycle management.
Business Intelligence (BI) is a technology-driven process of analyzing and presenting business data to support data-driven decisions and strategic planning. It involves using data analysis tools and methods to transform raw data into meaningful insights, dashboards, reports, and visualizations, helping companies identify trends, patterns, and opportunities.
Big data goes beyond the capabilities of traditional approaches, involving vast and complex datasets with characteristics such as volume (huge amounts of data), velocity (high speed of data generation and collection), and variety (different types of structured and unstructured data). Big data analytics and supply chain management employ advanced methods to extract valuable information and insights from this massive volume of data.
The Most Popular Database Management System Types Used in Business
Here is a list of the most widely used database model used in business:
Relational Database Management System (RDBMS) | Oracle, MySQL, Microsoft SQL Server, PostgreSQL, IBM DB2. |
Non-Relational Database Management System (NoSQL) | MongoDB, Cassandra, Redis, CouchDB, HBase. |
Document-Oriented Database System | MongoDB, CouchDB. |
Graph Database System | Neo4j, ArangoDB, OrientDB. |
Key-Value Database Management System | Redis, Memcached, Aerospike. |
Successfully employing a manufacturing data model helps businesses adapt to rapidly changing market conditions. Enterprises that consciously invest in these concepts and leverage data wisely can better understand their customers, optimize business processes, and make informed decisions based on industry datasets.
Business data systems are interconnected with modern business components and business analytics and data governance. Their utilization enables companies to be more agile.
Explore Top Virtual Data Room Providers at Data-Rooms.org
In today’s digital age, securing sensitive data is paramount for businesses of all sizes. Virtual Data Rooms (VDRs) have emerged as essential tools for secure data storage, sharing, and collaboration, especially during mergers, acquisitions, due diligence processes, and other confidential transactions. Choosing the right Virtual Data Room provider is crucial for ensuring the safety and integrity of your data.
At https://data-rooms.org/, we understand the importance of finding a reliable VDR solution tailored to your specific needs. Our platform offers a comprehensive and up-to-date list of top Virtual Data Room providers, each carefully evaluated to help you make an informed decision. Here’s why you should consider exploring our recommended providers:
- Robust Security: Protect your data from breaches and cyber threats.
- Effortless Collaboration: Enable real-time document access and editing from anywhere.
- User-Friendly Interface: Streamline document management with intuitive designs.
- Compliance and Support: Providers adhere to industry standards and offer 24/7 assistance.
Visit us to make an informed choice and secure your business transactions effectively.